Accurate solar power forecasting is critical for grid stability, energy management, and maximizing renewable energy adoption. However, newly installed solar plants face a significant hurdle: limited historical data to train reliable forecasting models. A groundbreaking study published in Applied Intelligence (2025) introduces an innovative solution using transfer learning and deep neural networks (DNNs) optimized with metaheuristic algorithms. This article breaks down the research, its implications for the renewable energy sector, and practical applications for solar plant operators.
The Challenge of Solar Power Forecasting
Solar energy’s intermittent nature complicates grid integration and energy planning. Traditional forecasting models rely on vast historical datasets, which new solar installations lack. Without sufficient data, predictions become unreliable, leading to inefficiencies in energy storage, grid management, and financial planning.
The study addresses this gap by leveraging transfer learning—a technique where a model trained on one task (e.g., solar radiation prediction) is repurposed for a related task (e.g., power generation forecasting). This approach reduces data dependency and accelerates model training, making it ideal for new solar plants.
How Transfer Learning Transforms Forecasting
Key Methodology
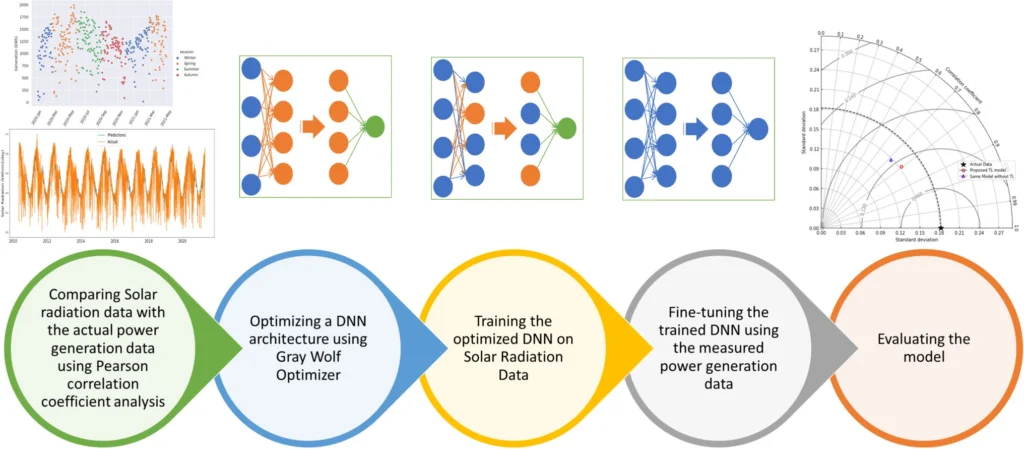
- Deep Neural Network (DNN) Architecture:
- A 3-layer DNN with 412-57-785 nodes was optimized using the Grey Wolf Optimizer (GWO), a metaheuristic algorithm inspired by wolf pack hunting behavior.
- The model was pre-trained on 11+ years of NASA solar radiation data (2010–2021) to capture global solar patterns.
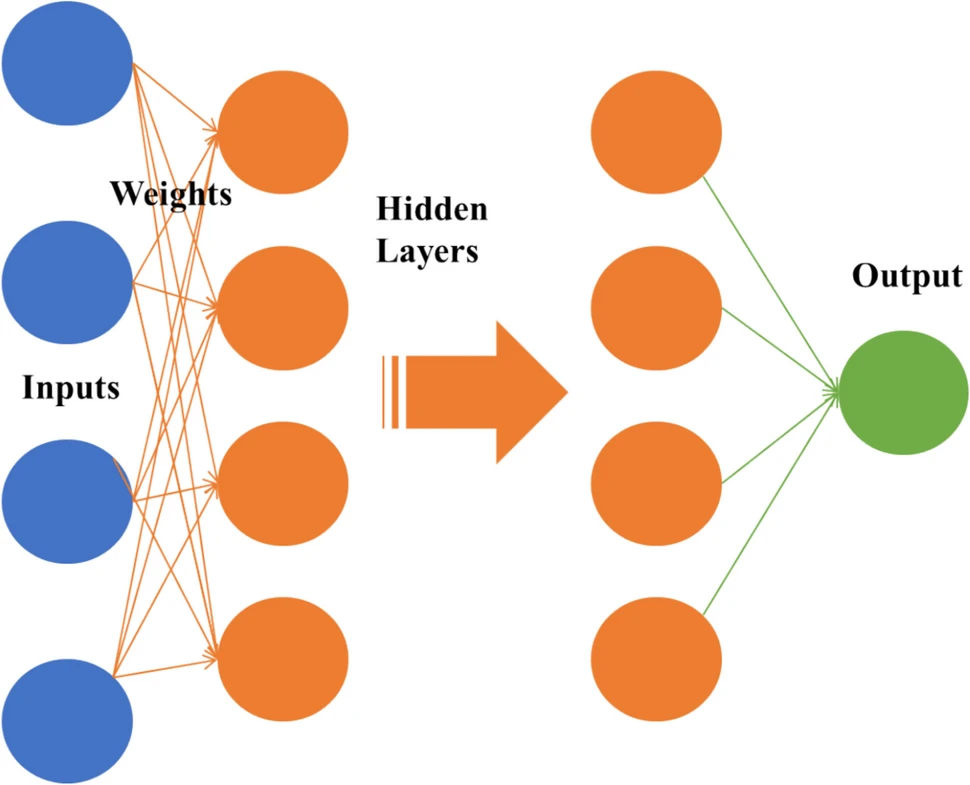
- Fine-Tuning with Plant-Specific Data:
- After pre-training, the model was fine-tuned using 487 days of generation data from a 400 kWp solar plant in India’s Himalayan region.
- Only 17 months of on-site data were used, demonstrating the method’s effectiveness with limited datasets.
- Performance Metrics:
- Mean Absolute Error (MAE) dropped by 19 kWh (173 vs. 192).
- R-value (correlation coefficient) improved by 7.7% (79.4% vs. 71.7%).
- Forecasting accuracy surpassed traditional models, even in volatile mountainous climates.
Case Study: 400 kWp Solar Plant in the Himalayas
The research focused on a grid-connected solar plant at Shoolini University, India, with the specifications below:
Parameter | Value |
---|---|
Location | Solan, Himachal Pradesh, India |
Capacity | 400 kWp |
Annual Solar Insolation | 5.15 kWh/m²/day |
Daily Generation (Avg) | 1,187 kWh |
Inverters Used | Delta Energy (10–50 kW capacities) |
PV Modules | Alpex Solar, 315 Wp polycrystalline |
Seasonal Variations:
- Spring: Peak generation (up to 1,984 kWh/day).
- Winter: Lowest output (as low as 15.2 kWh/day).
- Autumn: Most stable generation with minimal fluctuations.
Solar Plant Generation by Season
Figure: Daily generation varies significantly across seasons due to weather and solar irradiance changes.
Why Transfer Learning Works
Correlation Between Solar Radiation and Power Generation
The study found a 71.5% correlation between solar radiation and power generation, enabling knowledge transfer from radiation models to generation forecasts. Predictors like temperature range and surface pressure also played critical roles.
Top Predictors | Impact on Forecast |
---|---|
Previous 4 days’ generation | 32% |
Max/Min Temperature | 28% |
Solar Radiation | 24% |
Humidity & Precipitation | 16% |
SHAP Analysis: Revealed that historical generation data and temperature metrics were the most influential inputs, while wind speed had minimal impact.
Advantages Over Traditional Models
- Reduced Data Dependency:
- Pre-training on NASA’s global solar radiation dataset eliminates the need for years of on-site data.
- Faster Training:
- The optimized DNN trained in 26 seconds on an RTX3050 GPU, making it feasible for real-time applications.
- Scalability:
- The framework can be adapted to other renewable systems, such as wind farms, with similar data constraints.
Practical Applications for Solar Plant Operators
- Energy Storage Optimization:
- Accurate forecasts enable better battery charge/discharge scheduling, reducing energy waste.
- Grid Management:
- Utilities can balance supply-demand more effectively, minimizing reliance on fossil fuel backups.
- Financial Planning:
- Predictable generation data aids in securing investments and negotiating power purchase agreements (PPAs).
Challenges and Future Directions
While the study’s results are promising, challenges remain:
- Data Quality: Sensor accuracy and transmission errors can skew forecasts.
- Model Complexity: Highly optimized DNNs may require specialized hardware for deployment.
- Human Factors: Maintenance activities or unexpected shutdowns aren’t captured in models.
Future Research:
- Integrating real-time meteorological data from on-site stations.
- Exploring hybrid models with convolutional neural networks (CNNs) for spatial-temporal analysis.
Resources and Further Reading
- NASA POWER Dataset: Access Solar Radiation Data
- IEA-PVPS Report: Global Solar Trends 2024
- Full Study: Tajjour et